Artificial Intelligence
Trends in AI Technology for Businesses in 2022

As Artificial Intelligence continues to gain traction, a wide variety of new tools and services are being made available across different industries. The Internet of Medical Things (IoMT) opens up a wealth of possibilities for utilizing AI, particularly in solving problems related to privacy and data protection. Additionally, AI-enabled solutions provide physicians with the ability to quickly and accurately diagnose patients. A diverse range of companies, from startups to well-established firms, are adopting AI-centric strategies. A key trend in AI technology for businesses in 2022 is the emergence of no-code AI platforms. These platforms enable smaller businesses to swiftly incorporate sophisticated technological functionalities into their products, dramatically reducing the time required for developing and maintaining AI infrastructures by over 90%. Another area where AI is making significant strides is in customer service, with the widespread adoption of AI chatbot technology. These chatbots are able to provide immediate assistance to customers, improving overall satisfaction and reducing the need for human intervention. As businesses increasingly seek to provide 24/7 support, AI chatbot technology is becoming an indispensable tool for streamlining customer interactions and solving inquiries in real-time. The integration of AI chatbot technology into existing customer service systems is expected to become a standard practice across industries in the coming years.
AlphaFold is a new AI tool
AlphaFold is an AI tool that predicts the three-dimensional structure of proteins. It was developed by DeepMind and is based on an AI model inspired by the human brain. The system uses a large amount of input data and uses hidden networks to predict the desired output. The algorithm feeds protein sequences as input. The predicted three-dimensional structures are then generated from the sequences.
Using data provided by scientists, AlphaFold can predict individual protein structures. However, it is not completely accurate and can trip up on edge cases. The tool is limited to individual proteins and does not have a comprehensive knowledge of protein-DNA interactions or protein-small-molecule interactions. Researchers hope that AlphaFold will lead to breakthroughs in biomedicine.
The most difficult part of making accurate predictions of protein structures is figuring out how individual proteins interact with other cellular players. AlphaFold was able to predict the independent folding units of a protein in a CASP competition, but many challenges remain. For instance, the human proteome is a complex mixture of many proteins, which have multiple domains.
AlphaFold is a multimer model published as open-source software by DeepMind. The software is based on their Colab notebook and is available in Python. Users can view structure predictions, including PAE, as an image. The raw data can also be downloaded as a JSON file. However, the custom format is not supported by existing software, so users are required to use Python to plot and analyse the data.
DeepMind’s Gato is a general-purpose AI system
The Gato general-purpose AI system was trained on a variety of datasets, including images and natural language sentences. It has the capacity to handle billions of words and images and is able to perform a variety of tasks. It can even manipulate physical objects and can converse with humans, albeit in limited ways. Gato was developed by researchers at DeepMind and has been used for several real-world tasks.
This model is a good first step, but it still needs work to achieve true general-purpose AI. A general-purpose AI system should be able to perform a wide variety of tasks, without the need for heavy hard-coding. Gato’s ‘context window’ limit is one of its biggest weaknesses. This ‘context window’ is the amount of information that a system can remember at one time. That’s why Transformer-based language models are not yet ready to write a book or an essay, because they tend to forget the most important details. This ‘forgetting’ problem is a common problem in machine learning and is a known Achilles heel of the technology.
The Gato AI system was tested on a variety of control tasks and its averages were compared with specialist agents. This gave Gato an opportunity to learn how to perform a variety of tasks and identify commonalities between them. The system performed okay overall in the tests.
Uber’s self-driving car crash
An investigation of Uber’s self-driving car’s deadly crash shows that the system failed to identify a pedestrian, bicycle, and jaywalker in its path, and failed to prevent the collision. Despite several attempts to detect Herzberg, the car was unable to avoid crashing into her.
Since the crash, Uber has halted its self-driving car testing program. The incident occurred near Tempe, Arizona, and was believed to be the first pedestrian fatality involving self-driving technology. It also raised questions about liability and the level of safety precautions required in an autonomous vehicle. As a result, Uber suspended its self-driving car testing program, but has now resumed testing at a reduced speed and with more restrictions. As of March 2018, there are no specific laws that govern the responsibility of companies involved in autonomous car accidents.
Uber is under fire for failing to properly train its drivers and implement its safety plan. The company also failed to enforce its anti-cell phone policy and failed to monitor its drivers’ behavior. The company should also make sure their vehicles are vetted by independent third parties to ensure their safety.
Uber’s self-driving car crashed into a pedestrian on March 18, causing her death. While Uber has not publicly released the details of the accident, the details surrounding the crash are important for the public. The crash occurred when the self-driving car failed to stop before it struck Herzberg.
MIT’s Task Force on the Work of the Future report
The MIT Task Force on the Work of the Future report outlines a set of recommendations to make MIT more sustainable and responsive to the evolving needs of society. Among its recommendations are the inclusion of social equity in the curriculum, experiential learning, and professional academic advisors as well as faculty members. Moreover, it calls for better preparation for graduate careers and opportunities for postdoctoral researchers. The report also calls for increased collaboration and international activities.
The Task Force on the Work of the Future is a multidisciplinary initiative involving more than twenty students and faculty members at MIT. Its mission is to identify constructive pathways forward as technology continues to improve the world of work. The Task Force’s work is grounded in scientific evidence and deep expertise in technology. In addition, it makes reasonable assumptions about future scenarios and develops policy-relevant ideas and insights.
The report cites examples of AI in the workplace, including the development of robots that can help workers perform complex tasks. It includes field studies that back up the MIT Task Force’s conclusions. In this way, the MIT Task Force on the Work of the Future report counters the common doomsday perspective that AI will eliminate jobs. AI will transform the way people perform their jobs, not destroy them.
The Work of the Future report outlines a future where there is enough work for everyone. Despite the fact that the US economy’s labor force participation rate is declining, human labor will be needed for the transition to the new economy. Consequently, it is critical to ensure that people are trained for this new environment.
Uber’s autonomous vehicle
Uber isn’t the only company interested in developing autonomous vehicles. The company also has a strong interest in them because it gets 75 percent of the fare rather than the usual 25 percent, and eliminating the driver would mean higher revenues for the company. Its future plans for autonomous vehicles include allowing customers to request driverless cars on demand, and positioning these vehicles throughout the world.
Currently, the company’s modified Volvo XC90 is driven on public roads by human drivers who help train the autonomous vehicle algorithm. They drive the vehicle on a set of roads to simulate the “perfect drive” and record data that helps the software drive in the pre-mapped areas. This data helps the algorithms learn how to drive in the pre-programmed area.
Ultimately, the deployment of the AVs is dependent on the success of the test program. It’s likely that Uber will continue testing in controlled environments until it can prove that the technology is reliable enough to drive in the real world. In the meantime, the company will continue testing its autonomous vehicles in Pittsburgh.
Uber began working on developing autonomous vehicles around 2015 and partnered with Carnegie Mellon University’s National Robotics Center. It poached 40 scientists and engineers from the university and formed the Uber Advanced Technologies Group (UTG) to develop self-driving technology. It has also hired hundreds of employees to develop the technology.
Uber’s self-aware AI
AI is a powerful tool for solving problems. It helps us cluster customers and understand their needs. By using machine learning and other methods, AI can make our journeys better. For example, Uber uses AI to understand its drivers and predict where they will end up. It also uses AI to detect fraudulent rides, match riders and drivers, and optimize routes. It can even handle customer service incidents and route them to the most appropriate agents. These AI systems have increased Uber’s efficiency and customer satisfaction by 10 percent.
Uber is testing its self-aware AI in real streets. Its modified Volvo XC90 is driven by humans in order to map the area and simulate a “perfect drive.” The data from these “perfect drives” helps the software learn how to drive within the mapped area.
The autonomous cars have not reached Level 4 of autonomy, but Uber is working on it. It has also revealed its plans to buy up to 24,000 Volvo XC90 SUV taxis. These robo-taxis have taken over 50,000 passengers on over two million miles in pilot projects. However, the companies are still not sure if they will roll out these robo-taxis nationwide.
A recent fatal accident in Tempe, Arizona, has highlighted the risks of self-driving cars. A recent report in Commentary blamed the pedestrian while a preliminary National Transportation Safety Board report blamed Uber’s self-driving AI for the fatal crash.
Artificial Intelligence
Phala Network & NeurochainAI Collaborate for Decentralized GPU Compute Access
Find out how the Phala Network and NeurochainAI partnership is revolutionizing decentralized GPU computing, unlocking new possibilities for your AI projects.
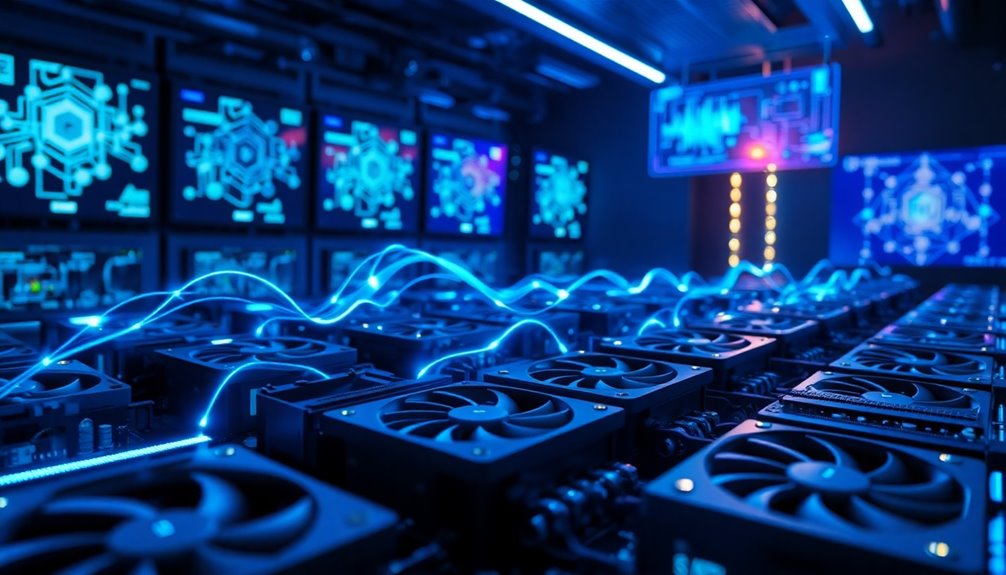
Phala Network and NeurochainAI are teaming up to transform decentralized GPU computing for AI projects. This collaboration taps into Phala's secure Trusted Execution Environments, giving you access to over 14,000 GPU providers for efficient and cost-effective AI development. You'll benefit from significant cost reductions and flexibility with a pay-per-use model, all while ensuring the security and privacy of your data. NeurochainAI's community-focused ecosystem enhances collaboration, making it easier for you to connect and innovate. As you explore this partnership, you'll uncover even more ways it could change the landscape of decentralized computing.
Key Takeaways
- Phala Network and NeurochainAI's partnership enhances AI development with secure, high-performance GPU resources using Trusted Execution Environments (TEEs).
- The collaboration provides access to over 14,000 GPU providers, reducing costs by up to 90% compared to traditional cloud services.
- NeurochainAI's ecosystem leverages community-owned GPUs for a decentralized AI compute environment, emphasizing data security and privacy.
- Decentralized GPU networks are set to drive innovation across sectors like healthcare and finance by offering scalable computational solutions.
- The integration of advanced security measures ensures safe processing of sensitive AI tasks within the decentralized framework.
Partnership Overview
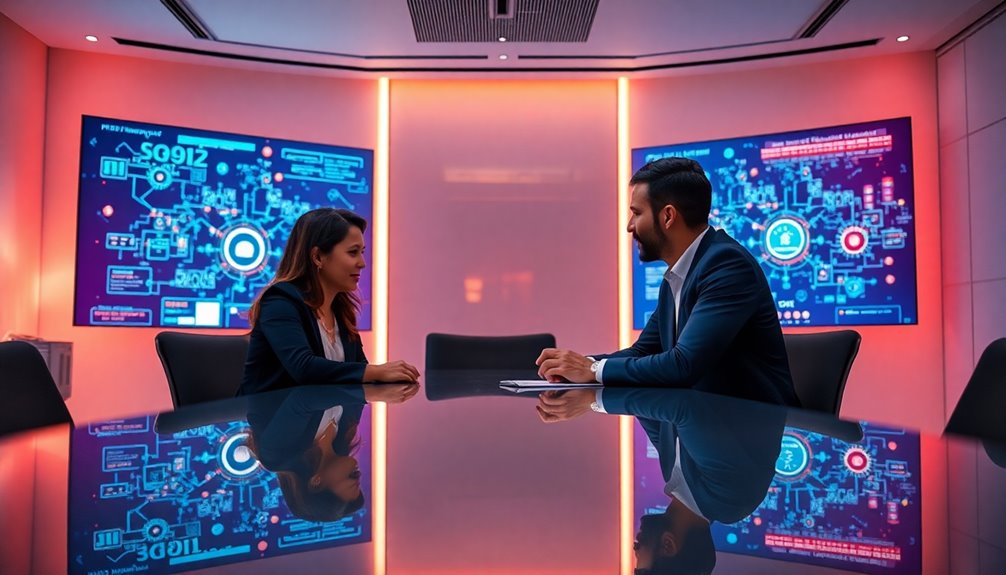
In a groundbreaking collaboration, Phala Network and NeurochainAI are joining forces to revolutionize the AI landscape. Their partnership aims to enhance NeurochainAI's ecosystem with secure, high-performance GPU resources, making advanced AI technologies more accessible and impactful. By combining their strengths, they're targeting the next generation of AI-driven solutions while emphasizing trustless computing and decentralized AI.
They'll utilize Phala's Trusted Execution Environments (TEEs) for secure GPU computations, leveraging its CPU and GPU TEE capabilities to propel AI development. This integration will allow them to combine Phala's secure GPU TEE solutions with NeurochainAI's infrastructure, ensuring that computations remain secure, privacy-preserving, and verifiable.
The benefits of this partnership are substantial. You'll gain access to scalable and cost-effective GPU resources, enabling developers and researchers to pursue their AI projects with enhanced efficiency and security. Together, they'll unlock critical features on NeurochainAI's roadmap, creating transformative opportunities for the future of AI-driven technology. This collaboration signals a new era in which innovation across both ecosystems can thrive, pushing the boundaries of what's possible in AI. Notably, the network includes over 14,000 GPU providers, reflecting the extensive resources available for AI development.
Phala Network's Contributions

Phala Network significantly enhances the collaboration with NeurochainAI through its cutting-edge contributions in GPU technology and Trusted Execution Environment (TEE) infrastructure. By utilizing Nvidia H100 and H200 GPUs, Phala powers TEE technology, ensuring that decentralized AI operations are secure and efficient. You'll benefit from the integration with io.net's cloud network, IO Cloud, which allows for decentralized AI while maintaining the highest security standards.
Their TEE infrastructure combines Intel's SGX and Nvidia GPU TEEs, creating a hybrid model that guarantees secure, isolated computations. This means that proprietary data sent for inference in large language models is protected through a chain of trust, involving on-chain registration and attestations. Additionally, this partnership aims to bolster TEE capabilities by extending access to powerful GPU resources.
With over 40,000 TEE CPU nodes in place since the mainnet launch in 2021, Phala supports a scalable solution for running complex AI models like LLaMA 3 and Microsoft Phi. This decentralized infrastructure not only maintains data privacy but also enables web3 developers to offload computations from smart contracts to Phala's secure off-chain network. All these contributions empower a broad range of applications, ensuring a robust foundation for the collaboration with NeurochainAI.
NeurochainAI's Ecosystem
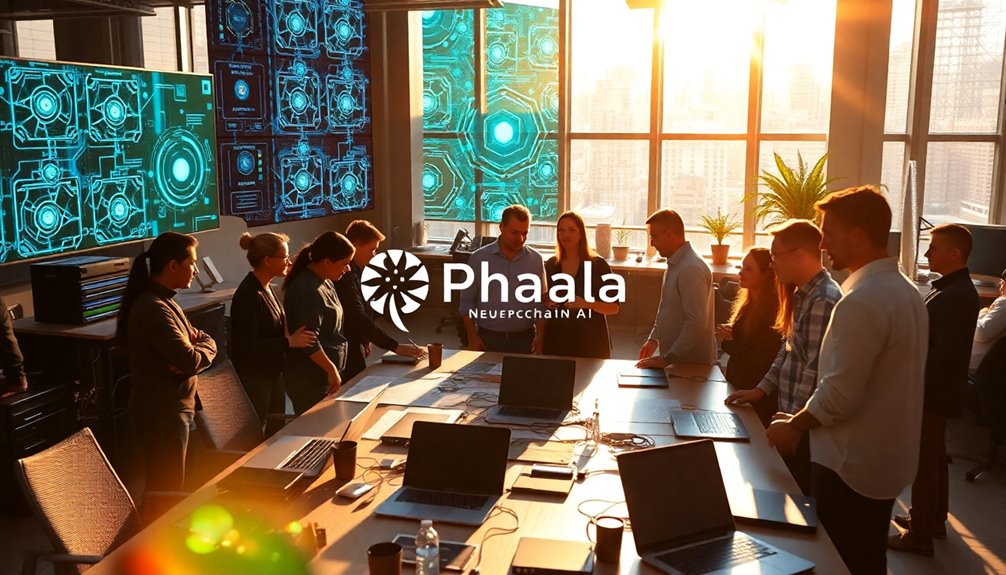
NeurochainAI's ecosystem thrives on a robust architecture that combines high performance with scalability, built on the innovative NCN Chain. This Layer 1 blockchain utilizes EVM and Optimistic Rollups, integrating both Layer 1 and Layer 3 solutions to enhance efficiency. With a hybrid consensus mechanism involving Neuron Validator Nodes and AI Mining Nodes, it ensures a seamless experience for users.
You'll find a decentralized AI compute environment that connects a global network of community-owned GPUs and NPUs, allowing for AI computations without the constraints of costly centralized cloud services. This setup promotes a community-driven approach to AI model creation, training, and deployment, leveraging a Decentralized Physical Infrastructure Network (DePIN) for optimal performance. Furthermore, the integration of AI and blockchain enhances the capabilities of this ecosystem, providing trust and intelligence to users.
Data security and privacy are paramount in this ecosystem. Community participation drives data validation, ensuring high-quality data while preventing unauthorized access. You can engage in transparent decision-making when it comes to AI development and usage.
Finally, NeurochainAI offers a decentralized AI as a Service (DAIAS) platform, featuring an AI model marketplace and tools for cross-chain interactions, all while encouraging community involvement through gamification and entertainment.
Benefits of Decentralized GPU
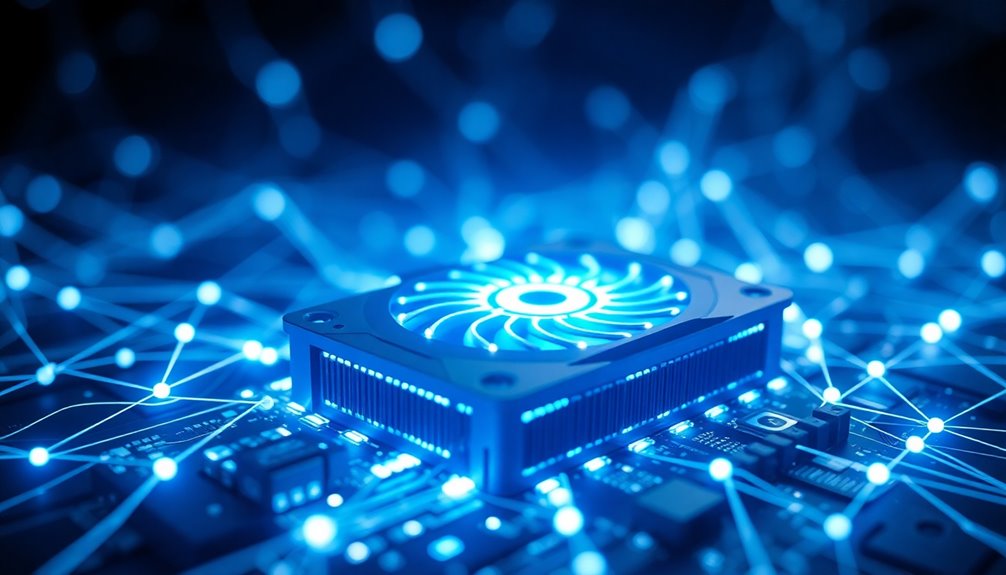
Decentralized GPU networks offer significant advantages, making advanced computational power accessible to a wider audience. First, you'll find that these platforms tap into underutilized GPU resources, allowing you to access powerful hardware at a fraction of the cost of traditional cloud services. With a pay-per-use model, you only pay for the resources you actually use, optimizing your expenditures without heavy upfront investments.
Moreover, the flexibility of decentralized GPU networks allows you to scale your resources dynamically based on demand. Whether you need to ramp up for a big project or scale down during quieter times, you can adjust your GPU usage accordingly. This efficiency is particularly beneficial for startups and individual researchers who may have fluctuating needs. Additionally, the demand for efficient GPU usage drives innovation in resource management, further enhancing the capabilities of these platforms.
Additionally, the accelerated processing capabilities of GPUs mean you can complete tasks in minutes that would take hours on CPUs. This speed boosts productivity, especially in AI, machine learning, and big data analytics. With blockchain technology ensuring transparency and security, decentralized networks democratize access to powerful GPUs, enhancing innovation and making advanced computing more accessible for everyone.
Technical Integration Details
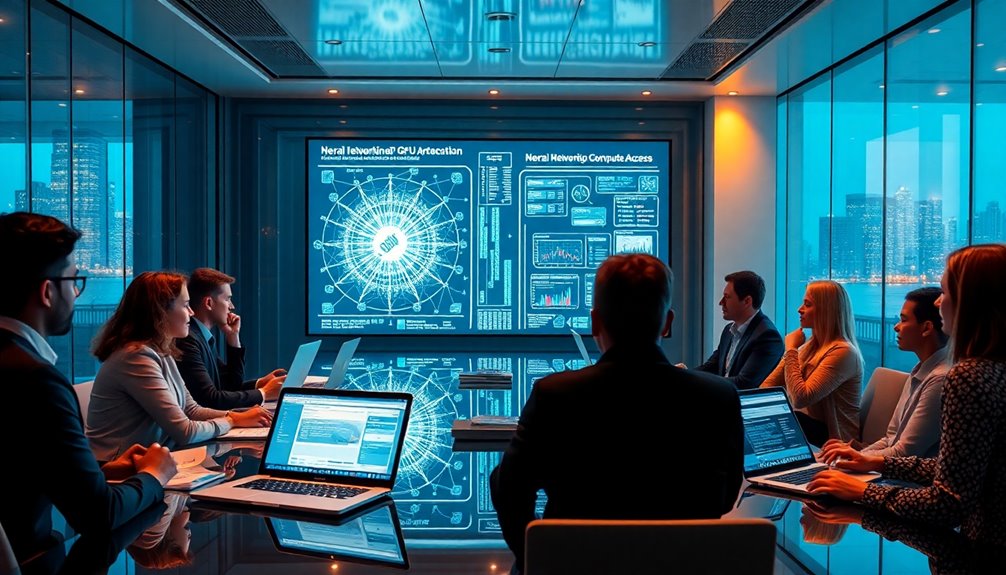
In this collaboration, the integration of Trusted Execution Environments (TEEs) plays a crucial role in enhancing security and privacy for computations. By utilizing Phala's robust CPU and GPU computing capabilities, you gain access to scalable and cost-effective resources tailored for AI development, machine learning, and computational research. With over 30,000 TEE CPU nodes and support for NVIDIA's H100 and H200 GPUs, you'll experience high-performance capabilities for training large AI models.
The platform ensures data confidentiality through advanced key management and state encryption, safeguarding the integrity of your AI models. You'll benefit from dynamic instance management, allowing seamless migration of workloads to secure instances if vulnerabilities arise. Moreover, Phala's blockchain-based attestation enhances the security of your computations.
With the IO SDK, integrating GPU resources is straightforward, enabling efficient resource allocation for your AI applications. The globally distributed GPU resources act like a CDN, bringing computation closer to end users. Additionally, you can engage with a community of over 14,000 GPU providers, accessing 200TB of GPU memory and a marketplace for quantized AI models, making this collaboration a comprehensive solution for decentralized GPU compute access.
Future Implications of Collaboration
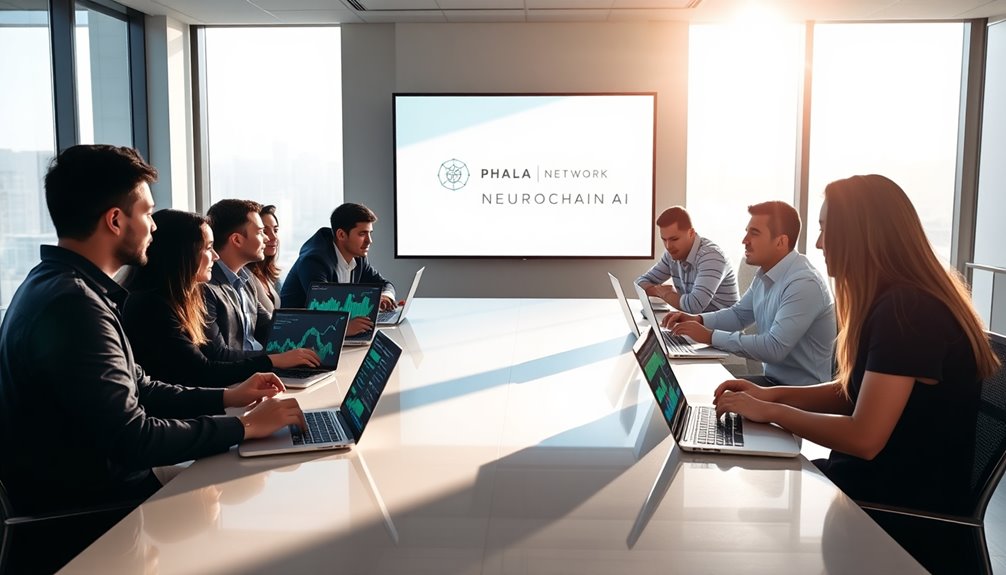
This collaboration is set to revolutionize the landscape of AI and machine learning by providing unprecedented access to scalable, cost-effective computational resources. You'll benefit from decentralized GPU networks that not only reduce costs—potentially saving you up to 90% compared to traditional cloud services—but also allow for customization to suit your specific needs. With access to powerful Nvidia H100 and H200 GPUs, you can tackle demanding AI tasks more efficiently.
Moreover, enhanced security features, such as Trusted Execution Environments, will ensure that your sensitive AI workloads are processed securely. This trustless computing environment will enable you to maintain data privacy while conducting verifiable computations, crucial in today's decentralized ecosystems. Additionally, the integration of Phala's GPU and CPU TEE computing will enhance your AI ecosystem, providing even more robust computational resources.
As the demand for decentralized GPU networks grows, industries like healthcare, finance, and autonomous technology will see significant innovation. The ability to scale and adapt quickly to changing demands will make these decentralized solutions even more appealing. Looking ahead, advancements in GPU architectures and AI optimizations will further enhance your capabilities, paving the way for a new era in AI development and deployment. Embrace these changes to stay ahead in a rapidly evolving technological landscape.
Frequently Asked Questions
How Can Developers Access Phala's GPU TEE Compute Resources?
To access GPU TEE compute resources, you'll need to integrate with the Phala Network's infrastructure. Start by connecting to their Trusted Execution Environment (TEE) nodes, which utilize high-performance Nvidia GPUs. Ensure your applications are designed to leverage these resources effectively. You can then deploy your decentralized applications securely, benefiting from the strong cryptographic protections that TEE offers, enabling you to handle sensitive workloads while maintaining data privacy and security.
What Types of AI Applications Can Benefit From This Partnership?
You can leverage this partnership for various AI applications, including accelerated training of deep learning models and processing complex neural networks. It's perfect for natural language processing tasks, enabling real-time language translation or generative AI. Computer vision projects, like high-resolution image analysis, also benefit significantly. With secure and scalable GPU resources, you'll find it easier to develop advanced technologies across diverse fields, enhancing performance and accessibility for your projects.
Are There Any Costs Associated With Using the Decentralized GPU Network?
Yes, there are costs associated with using a decentralized GPU network. However, you'll find that these costs are structured in a way that's often more affordable than traditional cloud services. You'll pay on a pay-as-you-use basis, avoiding hefty upfront investments in hardware. Plus, you won't deal with complex infrastructure management, making it easier for you to access and deploy GPU resources when you need them, all while potentially saving on operational expenses.
How Does This Partnership Ensure Data Privacy for Users?
This partnership ensures your data privacy by employing advanced cryptographic techniques and Trusted Execution Environments (TEEs). Your transactions and personal information get encrypted, preventing unauthorized access. Only authorized parties can access your data, keeping it confidential throughout the computation process. With verifiable computations and smart contracts, you can trust that your sensitive information remains protected. The decentralized nature of this system further reinforces the integrity and security of your data.
What Is the Expected Timeline for Feature Rollout in Neurochainai?
You can expect the feature rollout for NeurochainAI to follow a structured timeline. Initially, there'll be an Alpha phase for internal testing, followed by a Beta phase for selected users. Each stage will last a few weeks, allowing time for feedback collection and adjustments. After evaluating test results and user feedback, a full rollout will occur, likely within a few months. Clear communication will keep you informed throughout the process.
Conclusion
In conclusion, the collaboration between Phala Network and NeurochainAI is set to revolutionize decentralized GPU compute access. By combining their strengths, they're not just enhancing computational power but also empowering developers and users alike with greater efficiency and security. This partnership opens up exciting possibilities for innovation in AI and blockchain, paving the way for future advancements. Keep an eye on how this synergy unfolds; it could redefine the landscape of decentralized computing.
Artificial Intelligence
5 Ugly Truths About Data—And How to Win at AI Regardless

There has been a significant amount of conversation and written material concerning what artificial intelligence can do, but without data, it simply remains an elaborate concept that seems more at home in the realm of science fiction. This blog will delve into various undeniable truths about data and will outline strategies through which you can achieve success with AI despite these facts.
Understanding the basics of data management enables your organization to reap incredible business value from using AI. And by arming yourself with the right knowledge, you can ensure that data is managed effectively and efficiently. So read on and learn how you can take your business to the next level with data management!
#1 Developers Need the Right Data to Build AI Applications
Data is the lifeblood of any business. Without good data, developers can’t build AI applications, and businesses can’t compete on a level playing field. Luckily, there are many ways to get more accurate and actionable data – from improving customer acquisition funnel quality metrics to building a better user experience. However, getting the data you need is not a trivial task.
Developers need the right data to build AI applications, but most businesses don’t have it. So, what can you do to win at AI regardless? The answer is simple – start collecting data today!
Understanding how Machine Learning Works Will Give You an Edge in The Race to Build Better AI
Machine learning is one of the most important aspects of AI, and if you want to build better applications, you must understand how it works. If you don’t have the right data, your machine learning will be inaccurate and ineffective – making your project a total failure. Getting access to high-quality data sets as early as possible in your AI project is crucial.
Once you have this valuable information, building more accurate, AI applications become much easier. Moreover, by understanding how machine learning works in detail, you can create better machines capable of making sophisticated decisions quickly and efficiently – something which could prove very beneficial for businesses across all industries.
Data Is the Lifeblood of AI Development
Data is the lifeblood of AI development; without it applications like artificial intelligence (AI) will be unable to learn and grow. To get your hands on as much data as possible, businesses are starting to see the importance of data collection.
The race is on – so whichever company gets their hands on the most accurate and up-to-date data will be in a better position to win in this digital age. Without quality data, AI applications cannot learn effectively or even exist at all!
Ensuring Data Quality Is Essential for Building Successful AI Applications
Building successful AI applications is all about getting the right data in the right format. This can be a challenging task, but with the help of appropriate cleansing and standardization techniques and trained machine learning algorithms, it’s possible.
If insufficient data leads to wrong decisions or inaccurate predictions, then it has disastrous consequences for everything from business operations to customer service. It is important therefore to ensure that your data meets high-quality standards in order not just to build accurate AI applications but also to safeguard your business’s long-term prospects.
Accuracy and timeliness are key to success
Accuracy and timeliness are two factors that are key to success when it comes to data-driven decision-making using AI. Without accurate data, your AI applications will make mistakes which can have big consequences for the business.
Make sure you have a clear understanding of how AI works so that you can use it effectively for your business goals. Invest in the right tools and processes – this will help speed up the process of getting accurate and up-to-date data.

You Can’t Fake Intelligence
It is essential to be honest and open when it comes to data. This will help you avoid making wrong assumptions or applying AI in a way that’s not fully justified. Another key point is having the right data – something that isn’t easily faked or manipulated.
Only by using accurate and up-to-date information can you effectively use AI tools. And remember, even if your data looks perfect on the surface, don’t forget that people are always capable of questioning it and seeing things in a different light! To really reap the benefits of artificial intelligence, we need to be open about how it works – so everyone can own its potential as well as critique its limitations.
#2 All Businesses Face Data Challenges
Data is essential for any business, but it doesn’t always come easy. The good news is that you can overcome data challenges and win at AI with the right tools and strategies. By understanding your business’ data needs and using the right tools, you’ll be able to improve employee productivity and customer engagement.
Finally, don’t be afraid to ask for help – there are experts available who can assist with your AI strategy. Armed with this knowledge, you can ensure that your business is ready for the future of data-driven technologies.
You Need Skilled Employees to Handle Data Responsibly
Data is power and must be handled with care if you want your business to succeed in the age of big data. Having a skilled data team can make the most out of analytics and uncover insights that would otherwise have been difficult or impossible to find.
Only by implementing AI-based cleaning will you be able to extract valuable information from your masses of unprocessed data. In short, having a well-rounded strategy for responsibly handling data is essential.
Your Data Strategy Needs to Be Tailored to Your Business
There is no doubt that data plays an integral role in the decision-making process for businesses. In fact, it can often be the deciding factor between success and failure. Without a data strategy, your business will struggle to stay afloat in today’s competitive market.
A sound data strategy starts with understanding your business – its goals and how best to achieve them through data-driven decisions. You need to know your target audience, their needs, and how you can reach them most effectively using digital channels. Once you understand these things well, creating robust plans that accurately reflect this information becomes much easier.
You Can’t Avoid Data Altogether
Data is a fact of life, and, as such, you need to learn how to manage it wisely. This involves understanding the limitations of AI so that you can prepare for the future when it comes to data usage in your business. By doing this, you’ll be able to maintain control over your operations and remain competitive against those without this knowledge. It’s also crucial to take action now – start making changes to your data management process! Doing so will help streamline operations and improve efficiency overall. In addition, by having sound analytics in place, you’ll be better equipped to understand customer behavior – an essential factor for any successful business today or tomorrow.
Data Isn’t Always Accurate or Up-To-Date
There is no doubt that data is an essential asset for the business. However, it’s not always accurate or up-to-date – making it a valuable item to protect and manage wisely. Access to accurate and up-to-date data allows you to make better strategic decisions in the marketplace. You’ll be able to identify trends more quickly, estimate customer behavior accurately, etc.
By using artificial intelligence (AI), you can easily clean and organize your data into formats that are easier for you to use and understand. This way, there’s less need for manual input leading to increased efficiency in your decision-making process overall!
Statistics Can Help You Defend Yourself Against Accusations of Wrongdoing
The use of data analytics can help you make sound decisions that will protect your business from accusations of wrongdoing or unethical behavior. It can also improve customer retention, engagement and growth rates, among other things.
Understanding the basics of statistics can help you see patterns in data that might otherwise go unnoticed. Doing so could save you a lot of time and money in the long run. Moreover, using them to defend yourself against accusations is an effective way to restore your reputation – something that’s often crucial for businesses striving for success.
#3 Managing Data Can Be Hard
Data is power. And if you want to be successful with AI, you need to be able to manage data well. That’s why it’s important to break data down into manageable chunks, use the right tools for the job, and analyze it regularly to identify trends and patterns. If you’re struggling with any of these aspects, don’t be afraid to ask for help from your Data Science team. They’re here to support and help you achieve your data management goals.
Be Prepared for Machine Learning Failures
Machine learning can be a powerful tool for businesses but comes with risks like anything else. You can do many things to minimize the chances of such failures, but some cannot be avoided altogether. For instance, if your data set is not big enough or improperly formatted, the algorithm will fail at recognizing patterns and making predictions.
Another common problem is over-fitting – in which the algorithm becomes so obsessed with its own findings that it erroneously generalizes from the data set and makes incorrect judgments. This might lead to bizarre or even dangerous decisions being made by machines – something that could prove costly for businesses in terms of money wasted on mistaken investments or damaged relationships.
Artificial Intelligence Can Help Make Sense of Your Data
Artificial intelligence (AI) can help make sense of your data and use it to provide insights that you may not have been able to find before. Using AI, you can extract valuable information from your data sets in a way never possible. AI can also suggest actions or strategies based on the data gathered thus far. In this way, you are better positioned to make informed decisions that would result in improved outcomes for your business.
Make Sure Your Data Is Accurate and Up to Date
Accurate data is key to success when using machine learning algorithms. If the data you are using does not reflect reality as it exists, the models will be skewed and inaccurate. For example, if your company sells products that require specific measurements to qualify for a discount, make sure all of your sales figures include these measurements so that the AI model can properly estimate whether or not customers meet eligibility requirements.
Inaccurate data can also lead to faulty predictions or unintentional wrong decisions being made by machines. Be sure to regularly check the accuracy of your data so that you can make informed decisions about how to use AI in the future.
Your Data Is Worth More than You Think
Data is one of the most valuable resources a business has. If you’re not using it to your advantage, you’re wasting precious assets. You can take a few steps to ensure that your data is as useful as possible:
- Recognize that data is more than just numbers and figures on a screen or paper. It’s information that can help you identify trends, analyze customer behavior, and improve marketing campaigns.
- Make sure all team members have access to the right data tools so they can use it effectively and efficiently. This includes IT experts and frontline employees who need to understand how their actions impact company performance (and vice versa).
- Guard against erroneous or incomplete data by regularly cleansing it, verifying its accuracy, and making changes only after thorough analysis confirms results are accurate (and meaningful). In other words – treat data like gold!
Make Sure to Track Your Data’s Progress
To make data-driven decisions, it is essential to track all performance metrics. This will help you understand your model’s progress, identify any issues, and correct them before they become big problems. Once your model is up and running, it’s essential to track its progress regularly to measure the results.
You need this information to adjust things, both during the training and implementation phases. In addition, being data-driven enables you not only build successful AI models but also trust them with critical tasks related to business operations.
#4 Data Is a Business Asset
Data is the lifeblood of any business, and you need to protect it vigilantly. No business can survive without data, and even those that do are at a disadvantage when competing with companies that have data at their disposal.
Here are 5 ugly truths about data that you need to know to win at AI:
- Your data is your business’s most valuable asset.
- You need to have a plan for how you’re going to manage and use data to keep it safe and competitive.
- The sooner you start taking steps to protect your data, the better off you’ll be.
- If your data is compromised, there’s nothing you can do to undo the damage.
- The sooner you understand and accept these truths, the better equipped you’ll be to manage and use data in your own business to win at AI.
You Need to Know What Data Is Worth
Data is important in any business – from big corporations to small startups. Without data, it’s difficult to manage or improve your operations. There are various data types, and each can be used for different purposes. For instance, customer data can be used for market research and creating new products, while sales data is essential for managing inventory and making informed decisions about pricing strategies.
Access to the right data type is crucial if your business grows sustainably and effectively. Automating routine tasks can free up time so that you can focus on more strategic initiatives. And keeping track of which data is worth collecting will help protect your valuable assets while growing them further!
Data Should Be Treated as A Precious Resource
Data should be treated like a precious commodity. Not only is it essential to have a data governance strategy in place, but making the most of data requires using AI technology for improved decision-making processes and automated data collection. To make the most out of your information resources, you need to ask smart questions that will give you insights that will help shape future course corrections.
Additionally, if your data isn’t well managed, it can lead to lost opportunities, inefficient customer service, and more. So do everything you can to keep track of this valuable resource – lest you find yourself at a disadvantage when trying to compete in today’s competitive market landscape!
Use Data Insights to Shape Your Business Strategy
Data is a valuable asset for any business and using data insights to shape your business strategy is essential. To do this effectively, ensure you use the right data analytics and machine learning tools. Doing so will help you improve decision-making processes, identify new opportunities, and optimize performance.
Above all else, don’t be afraid to make data decisions – it’s your company after all! And by utilizing big data and artificial intelligence technologies in predictive analysis and forecasting models, you can increase the accuracy of your predictions while reducing risk.
#5 The Power of AI Relies on Data
Data is the fuel that powers the engine of AI. Without it, these powerful engines will stall and you’ll be at a disadvantage when competing in today’s digital world whether a small business or a large corporation, getting access to the right data is crucial for success.
- Data is power – and you need access to it to succeed.
- Data is abundant – but it’s also vulnerable. Hackers and thieves are always looking for ways to steal it.
- Data hacking is a big business – and it’s increasing. You’ll be at a disadvantage if you’re not prepared for this.
- AI techniques are key to success – and you should use them to your advantage if you want to win in the data age.
- Data is an abundant resource – and it’s worth investing in to get the most out of it.
Collecting and Cleaning Your Data
No business would be able to function successfully without data. However, collecting and managing the right data can be daunting for small businesses or startups. There are various ways in which you can collect data – through surveys, interviews, or visits to your customer’s homes.
Once you have this information, it is essential to clean and analyze it correctly so you can unlock potential uses for AI applications. Ensuring that your data is up-to-date is essential for making informed decisions about future courses of action. By doing all these things effectively, you will gain an edge over your competitors who might not have as strong a grasp on their business analytics
Understanding the Importance of Data
Data is one of the most important tools in an AI arsenal. Without it, computers would be unable to learn or make decisions – something they, unfortunately, can’t do on their own! Everything you know – from what foods you like to how successful your ventures have been so far – is based on data.
And if we want machines to become more innovative and better at making critical judgments, we need more and better data. Luckily, there are various ways businesses can collect quality data- even if it’s not always easy. By using machine learning algorithms wisely, organizations can reap huge benefits regarding efficiency and effectiveness in analytics.
Taking Advantage of Smart AI
There is no doubt that the advancement of intelligent AI is profoundly impacting our lives and day-to-day tasks. From improving our daily routines to making decisions based on data, technology has come up with innumerable ways in which it can be put to use. However, without accurate data, AI cannot function properly. So businesses need to take measures towards collecting and managing their data effectively.
Doing so would help you better understand your customers, learn more about your industry trends, and act faster when adopting new technologies or strategies. Apart from using data analytics for strategic decision-making purposes, you should also implement artificial intelligence (AI) into your business processes – particularly customer engagement and marketing initiatives. Doing so can accelerate the process while ensuring that all information collected is systematically analyzed for future benefits!
Using Machine Learning for Predictive Analytics
Machine learning is a field of computer science that allows computers to learn from data independently. This can be used for a number of purposes, such as predictive analytics (the ability to make predictions about future events based on historical data). Without the correct data, you can’t train your machine learning models, which will ultimately limit its capabilities.
As such, it’s essential to have a sound data management plan in place so that all your relevant information is always accessible. Doing so will also give you more significant insights into how customers behave and help improve customer service levels and engagement rates.
Analyzing your data with AI tools
Understanding your customers is essential for any business. However, with the help of AI tools, this task becomes a lot easier. Predictive analytics enables you to identify customer trends and predict their behavior in the future.
This can help you make informed decisions about what products or services to offer and thus significantly improve your bottom line. However, data analysis has always associated risks – be sure to understand these before proceeding! Otherwise, you risk making wrong assumptions which could adversely affect your business operation in some way or another.
Be Mindful of Potential Dangers Posed by Artificial Intelligence
While data-driven decision-making could be a huge boon for businesses, there are also some risks. By generalizing from their limited dataset, machines may make incorrect judgments that can have costly consequences for companies. For example, if a machine were designed to predict sales trends and began making predictions based on current customer sentiment rather than actual sales figures, this error would quickly become apparent and lead to disastrous results. It is important to stay vigilant when using AI tools and ensure that all data is verified adequately before making any decisions.
Frequently Asked Questions
Artificial intelligence won’t necessarily make us smarter or even more efficient than we are today.
Although AI is often touted as a potential savior for humanity, the reality is that it’s only making things worse for us. Here are five ugly truths about data that we all need to be aware of in order to combat artificial intelligence: First, AI is exacerbating existing inequalities and biases, rather than working to eliminate them. Second, AI has the potential to displace human workers and reduce the availability of jobs. Lastly, AI is also fueling the surveillance state, giving governments and corporations unprecedented power to monitor and control individuals. To combat these challenges, it’s crucial for us to have an overview of atlas of ai and understand how AI is being used and its implications for society. By staying informed and critically evaluating the use of AI, we can work towards minimizing its negative impacts and harnessing its potential for good.
- Data manipulation occurs on an industrial scale, with corporations profiting from our personal information.
- Personal data can influence and manipulate people’s opinions, emotions, and behavior.
- Despite the widespread belief that AI has made us smarter, data shows that humans still outperform machines at various tasks.
- As machine learning gets better at recognizing patterns in vast amounts of data, Big Brother will become even more powerful than he already is. Meaning governments and other entities will have access to immense troves of personal information.
- Artificial intelligence only makes things worse for ourselves as it progresses and becomes more sophisticated.
Big data doesn’t mean big insights.
Before using big data in your business, you need to consider a few factors. Firstly, big data is often inaccurate. This means that the data may not accurately reflect the real world and can slow decision-making. Second, big data can cost a lot of money and time to use. You may need to hire specialists, purchase expensive software, or spend lots of time processing and analyzing data. Third, we don’t understand all the uses for big data yet. There are many potential applications that we haven’t even begun to explore. Fourth, big data has privacy implications. By collecting vast amounts of data, companies can track our every move and collect sensitive information about us. fifth, big data doesn’t always lead to better insights. Sometimes it’s simply overwhelming and confusing, and it would be easier to get better results by focusing on a smaller number of data sources.
All data is biased.
Yes, data is biased. But that doesn’t mean we can’t use it to make sound decisions. The key is to use multiple data sources, understand how different algorithms work, and carefully select which models or data sets are used in a given decision-making process. Even the most careful data analysis is bound to include some level of bias, but with the right tools and techniques, you can mitigate it as much as possible. To achieve good insights and make sound decisions, you must be aware of your biases and work on accounting for them.
We need to be careful not to rely too heavily on AI – it could do more harm than good in the long run
Yes, AI has many benefits for business operations and decision-making processes, but we need to be aware of its potential flaws to make intelligent choices. At this point, AI has the power to change and disrupt many industries, including finance, healthcare, transportation, retail and more. While there are immense benefits to using AI for these purposes, we need to be careful not to rely too heavily on it – this could actually do more harm than good in the long run. There is a growing concern that too much reliance on AI could lead us down the wrong path – one that may cause unforeseen damage or even lose our jobs altogether. So while AI has tremendous potential, we need to use it responsibly in order to avoid any potential negative consequences.
Do we need a “data czar” to win at AI?
Yes, there is a need for a data czar to help companies manage data effectively. This person will have the skillset and experience necessary to lead organizations through a data-driven transformation. A data czar refers to someone with deep knowledge and understanding of data. This person will be instrumental in helping companies make informed decisions about data-driven initiatives, such as expanding their customer base, developing new products and services, or even increasing revenue. In short, having a data czar is essential in helping companies win in the digital age, where information is power and having all the right information can mean the difference between success and failure.
Machine Learning Is a False Prophet
In general, machine learning is a technique that allows computers to learn from data sets on their own. This can be used for a variety of purposes, such as recognizing images or words, making predictions, or even controlling machines. Currently, however, many experts remain unconvinced of the full potential of machine learning. They believe that many problems still need to be solved before this technology can completely replace humans. However, as machine learning gets smarter and more ubiquitous, humans will need to learn how to cooperate with machines in order not to be replaced altogether. In addition, as humans are better positioned to make ethical decisions when it comes to big data due to their ability to empathize with the consequences of their actions, it is likely that machine learning will benefit society in the long run.
How can we best use data in our businesses?
There’s no doubt that data is the lifeblood of any business – and it’s important that we use it in the right way to make informed decisions. So how do you go about doing this? Start by taking a holistic view of your data. This means understanding all aspects – from sales and marketing channels, to customer service and product development – to create a complete picture of what’s happening. Then use analytics Only if it gives you a clear understanding of what is happening in your business. However, don’t be fooled by analytics – it can often paint an inaccurate picture of what is really going on. So always apply your own critical thinking skills when interpreting the data.
So what do you need to know about data if you want to win at AI?
If you want to win at AI, you need to retain as much data as possible. This data can be analyzed and used by your AI in order to learn and grow. Furthermore, make sure you’re not blindly trusting machine learning algorithms – understand how they work, why they’re advantageous for your business, and what could go wrong. Finally, be proactive in understanding the implications of big data on your company culture – confidentiality policies, HR strategies…you get the idea!
What should we be doing now to prepare for the future of AI and data?
You must stay aware of the trends in AI and data to take proactive measures when they happen. For example, are there certain areas of your life where machine learning is making better decisions than you? If so, what can you do to ensure that your data is used fairly and ethically? In addition, the future of AI and data raises some critical questions about who will control this information and how it will be used. Right now, we rely heavily on data to function every single day. However, as machine intelligence continues to develop, who will have access to this data and how will it be used? This is an area that requires much more research and discussion to conclude.
What are some of the biggest myths about data and how to dispel them?
Some of the most common myths about data and how to dispel them are as follows:
- Data is an enemy – The data you collect about your customers and employees can be used against them in various ways.
- You don’t need data – Data is essential for understanding your customers and business processes.
- You can’t make good decisions with data – By understanding how data works, you can make better decisions for your company and products.
- You have to be a data scientist to use data – Anyone can use data by understanding the basics of how it works.
- You need expensive software or hardware to collect data – There are many affordable ways to collect data using devices like smartphones and computer sensors.
Conclusion
So, you’re a data-driven business. That’s great! But do you know what data you need to build AI applications? And more importantly, do you have access to that data? The answer is a big ‘yes’, and here are the five ugly truths about data that will help you win at AI regardless. Read this blog and take action to access the data you need to build successful AI applications!
Artificial Intelligence
Deloitte’s AI Expertise and Partnerships With NVIDIA

Deloitte prides itself on its deep knowledge and expertise in artificial intelligence (AI), identifying unique challenges across different sectors and industries. This positions them perfectly to guide organizations into the advanced world of AI. Thanks to Deloitte’s in-depth understanding of AI, they are fully prepared to support your swift transition into this area. We explore the company’s partnerships with Nvidia and the AI Academy.
Deloitte’s AI Academy
Deloitte’s AI Academy will train aspiring professionals to become AI-fluent. It will also offer fellowships for doctoral research in AI and advanced analytics. These online learning courses will empower citizens and talent pools to embrace digital transformation and AI. The AI Academy will open to students in the U.S. and India, as well as international talent pools.
The AI Academy will cover topics such as data privacy and the design of AI systems. It will also provide training in MLOps. However, this will not be a self-paced curriculum, and you cannot complete it in your free time. Ultimately, this AI training will help you succeed in a fast-paced AI industry.
The collaboration between Deloitte and IIT Roorkee is aligned with the government’s “Digital India” vision, which aims to create a knowledge-based society with higher levels of AI proficiency. This initiative will help build the next generation of Indian talent and provide them with industry-relevant skills. Additionally, the two organizations hope to create a roadmap for the future of AI and machine learning in India.
In addition to providing AI training, the academy will connect AI practitioners with tools, knowledge, and infrastructure. Its training will be tailored to the needs of companies and individuals working in AI-intensive industries. Participants in the AI Academy will gain technical data skills, the fundamentals of Trustworthy AI, and a comprehensive understanding of AI across industries.

The AI Academy’s AI fluency sprints are designed for business leaders. They’re designed to fit around busy work schedules, and help leaders become more comfortable with AI. A new AI program is expected to create 97 million new jobs by 2025. But the problem with AI adoption is that most people don’t have the knowledge and skills to succeed. Luckily, the AI Academy has helped address this issue by creating a self-paced, online course that teaches the basics of AI while helping leaders succeed in their field.
“It’s our responsibility as a society and as business leaders to develop new talent with AI skills – not only for the engineers and data scientists, but also for every role in an organization, no matter how technical,” said Dan Helfrich, chairman and CEO, Deloitte Consulting LLP. “Through the Deloitte AI Academy we are endeavoring to develop future leaders with a higher level of AI proficiency for the benefit of our clients and society at large.”
The Deloitte AI Academy collaborates with the Deloitte AI Institute to offer learning and training that supports the Institute’s mission of enabling engaged conversations and innovative research for the positive development and growth of AI.
Its work with clients
Deloitte is a global provider of consulting, audit and assurance, financial advisory, and risk management services. Its member firms operate in 150 countries, and four out of five of the Fortune Global 500 companies are Deloitte clients. In order to stay competitive, they help clients develop and deploy data-driven strategies.
The company’s ReadyAI capability provides clients with scalable and flexible AI solutions that enable businesses to leverage artificial intelligence and machine learning for business transformation. ReadyAI replaces existing piecemeal solutions that can be costly and time-consuming. It helps clients identify anomalies and extract valuable insights from vast data. Deloitte’s ai capabilities can help clients gain competitive advantage and protect against fraud.
The company is also helping clients create AI solutions that can be used to tackle complex business problems. One example is a major integrated health care provider that had a manual invoice processing process. This jeopardized the relationships with suppliers and the supply of medicines. Deloitte’s AI solutions helped this organization eliminate its backlog of unpaid invoices and increased staff efficiency by 200%. In addition, the company developed its own platform that enables clients to access information across a variety of data sources.
Another Deloitte AI offering, Unlimited Reality, is helping clients capitalize on the next massive wave of digital technology. The company’s Deep Learning Platform uses the NVIDIA Omniverse Enterprise platform to develop virtual worlds and 3D simulations. The studio also provides a physical and virtual innovation space that helps clients explore AI use cases.
Another recent initiative is the Deloitte AI Academy. The academy’s purpose is to educate the next generation of AI professionals and expand the talent pool. It has recently launched a pilot program in India and plans to train 10,000 people in the United States within the next four years. Together with its AI Institute, the academy will promote the use of AI in businesses.
Its AI Dossier
The AI Institute at Deloitte recently released a report called “The AI Dossier” that explores business use cases for artificial intelligence. The report covers six different industries and highlights some of the most compelling uses of AI for business. It also explores some of the key business issues and opportunities that will arise as companies begin to use AI in their organizations.
A new initiative to foster AI research has been launched between Deloitte and UMD’s Smith School of Business. It is a partnership that aims to expand student and faculty research and learning opportunities. This initiative is designed to leverage the University of Maryland’s growing prominence in the field of artificial intelligence. Both organizations have a track record of working with AI, including joint research with Smith Analytics Consortium.
Deloitte’s AI institute will focus on building partnerships in the ecosystem around AI. It will provide case studies and insights from a variety of industries in order to ignite conversations about AI applications. It will also offer AI consulting services to clients and help them choose the right approach for their business.
A partnership between Deloitte and Nvidia has allowed the U.S. Postal Service to use vision AI to make better decisions. It also enables the company to improve customer service processes and interactions. It will increase efficiency and convenience. By leveraging machine learning and artificial intelligence (ML), AI will help companies improve their customer experience and service. AI can also help people with chronic diseases. By leveraging ML, AI can recommend the best clothing for their bodies, and wearable devices can monitor and provide real-time feedback.
Another new innovation that will help companies reap the benefits of AI is Unlimited Reality. This new service equips executives with the skills to exploit the emerging opportunities offered by virtual worlds. It also helps companies transform their industrial operations. Through it, businesses can monetize digital assets, engage customers with Web3 architectures, and optimize new workplace models. Similarly, it can help organizations improve the quality of their data.
Its partnerships with Nvidia
Deloitte and Nvidia have expanded their partnership to help enterprises create and deploy hybrid cloud solutions. Deloitte will provide clients with access to NVIDIA Omniverse enterprise platforms and AI platforms to develop and deploy cutting-edge tools and applications through their alliance. Deloitte will also provide clients with NVIDIA DGX A100 programs to enable 3D design collaboration and digital world simulation.
The companies’ partnerships are centered on building cloud-based AI and advanced visualization solutions. The firm is already a member of the NVIDIA Partner Network and has been recognized as a global leader for its commitment to building a cutting-edge AI practice. The partnership between Deloitte and Nvidia is expected to benefit both companies’ clients and employees.
NVIDIA Metropolis provides developers with access to a cloud-based environment optimized for AI development and data analytics. NVIDIA clients can leverage visual data and AI through this platform to improve operational efficiency, security, and IoT AI devices. In addition, NVIDIA Metropolis’ developer ecosystem provides an easy-to-use environment that can scale to meet a variety of needs.
With the power of AI, businesses and organizations can improve the way they interact with their customers. Wearable devices that capture and analyze data can monitor health and well-being, providing real-time recommendations. By analyzing millions of data points, self-learning AI systems can detect signs of disease and prevent continual illness. This is just one of many ways that AI and technology are affecting our health and well-being. Deloitte and Nvidia’s partnerships with Nvidia will help industries and governments leverage these technologies to make their processes and interactions more efficient.
About Deloitte
Deloitte provides industry-leading audit, consulting, tax and advisory services to many of the world’s most admired brands, including nearly 90% of the Fortune 500® and more than 7,000 private companies. Our people come together for the greater good and work across the industry sectors that drive and shape today’s marketplace — delivering measurable and lasting results that help reinforce public trust in our capital markets, inspire clients to see challenges as opportunities to transform and thrive, and help lead the way toward a stronger economy and a healthier society. Deloitte is proud to be part of the largest global professional services network serving our clients in the most important markets. Building on more than 175 years of service, our network of member firms spans more than 150 countries and territories.
-
Bitcoin3 weeks ago
How Likely Is Bitcoin to Hit 200K
-
Cryptocurrency3 weeks ago
Is MicroStrategy’s Bitcoin Gamble a Ticking Time Bomb? Anthony Pompliano Weighs In
-
Cryptocurrency3 weeks ago
AI Crashes the Bitcoin Party: Meet the Hyper-Intelligent Bots Trying to Snatch Your Slice of Crypto Glory
-
Cryptocurrency1 week ago
Analysts Spot AI Altcoin That Could Rally 30,000% by Q1 2025
-
Cryptocurrency3 weeks ago
Bitcoin’s Gold-Killer Moment: Is This the Mind-Blowing Rally to $120K That Shatters All Expectations?
-
Cryptocurrency3 weeks ago
XRP LEAKED: Ripple’s SEC Victory Could Send This Coin To The STRATOSPHERE
-
Cryptocurrency1 week ago
Dogecoin vs. XRP vs. RCO Finance: The Ultimate 2025 Profit Showdown
-
Cryptocurrency3 weeks ago
Ripple’s Bold 1% Pledge: The Daring Social Impact Move That Could Redefine Crypto’s Moral Compass